Prof. Dr. Josif Grabocka
Automatisiertes maschinelles Lernen ist der Hauptschwerpunkt des Machine Learning Labs. Das Team entwickelt hochmoderne Methoden, um Hyperparameter von Deep-Learning-Modellen zu optimieren – beispielsweise für große Sprachmodelle, generative Modelle, bestärkendes Lernen und neuronale Netze für tabellarische Datensätze. Dazu evaluieren wir Meta- und Transfer-Learning-Ansätze und nutzen Gray-Box-Optimierungsstrategien. Zudem konzentrieren wir uns auf vertrauenswürdiges maschinelles Lernen, wobei wir Robustheit, Fairness, Energieeffizienz und Interpretierbarkeit als zusätzliche Optimierungskriterien für Deep-Learning-Modelle berücksichtigen.
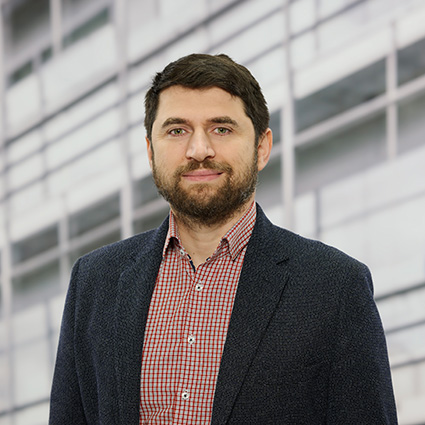
Prof. Dr. Josif Grabocka
Professur für Machine Learning
Aktuelle Forschungsprojekte
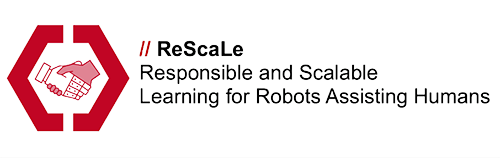
ReScaLe: Responsible And Scalable Learning For Robots Assisting Humans,
Carl-Zeiss Stiftung, 2022 – 2028;
Abgeschlossene Forschungsprojekte
Industriekooperationsstipendium: Automatisierte KI, Eva Mayr-Stihl Stiftung, 2019-2022
Ausgewählte Publikationen
-
Gresa Shala, André Biedenkapp, Pierre Krack, Florian Walter, Josif Grabocka
Efficient Cross-Episode Meta-RL
International Conference on Learning Representations (ICLR 2025) -
Rhea Sanjay Sukthanker, Arber Zela, Benedikt Staffler, Samuel Dooley, Josif Grabocka, Frank Hutter
Multi-objective Differentiable Neural Architecture Search
International Conference on Learning Representations (ICLR 2025) -
Arlind Kadra, Sebastian Pineda Arango, Josif Grabocka
Interpretable Mesomorphic Networks
Neural Information Processing Systems (NeurIPS 2024) -
Gresa Shala, Sebastian Pineda Arango, Frank Hutter, Josif Grabocka
HPO-RL-Bench: A zero-cost benchmark for HPO in Reinforcement Learning
International Conference on Automated Machine Learning (AutoML 2024)
Runner-up Best Paper Award -
Sebastian Pineda Arango, Fabio Ferreira, Arlind Kadra, Frank Hutter, Josif Grabocka
Quickly Learning Which Pretrained Model to Finetune and How
International Conference on Learning Representations (ICLR 2024) -
Arlind Kadra, Maciej Janowski, Martin Wistuba, Josif Grabocka
Scaling Laws for Hyperparameter Optimization
Neural Information Processing Systems (NeurIPS 2023) -
Sebastian Pineda Arango, Josif Grabocka
Deep Pipeline Embeddings for AutoML
ACM SIGKDD Conference on Knowledge Discovery and Data Mining (KDD 2023) -
Abdus Khazi, Sebastian Pineda Arango, Josif Grabocka
Deep Ranking Ensembles for Hyperparameter Optimization
International Conference on Learning Representations (ICLR 2023) -
Gresa Shala, Thomas Elsken, Hadi Jomaa, Frank Hutter, Josif Grabocka
Transfer NAS with Meta-Learned Bayesian Surrogates
International Conference on Learning Representations (ICLR 2023) -
Gresa Shala, Andre Biedenkapp, Frank Hutter, Josif Grabocka
Gray-Box Gaussian Processes for Automated Reinforcement Learning
International Conference on Learning Representations (ICLR 2023) -
Martin Wistuba, Arlind Kadra, Josif Grabocka
Supervising the Multi-Fidelity Race of Hyperparameter Configurations
Neural Information Processing Systems (NeurIPS 2022) -
Ekrem Öztürk, Fabio Ferreira, Hadi Jomaa, Josif Grabocka, Frank Hutter
Zero-shot AutoML with Pretrained Models
International Conference on Machine Learning (ICML 2022) -
Samuel Müller, Noah Hollmann, Sebastian Pineda Arango, Josif Grabocka, Frank Hutter
Transformers Can Do Bayesian Inference
International Conference on Learning Representations (ICLR 2022) -
Sebastian Pineda Arango, Hadi Samer Jomaa, Martin Wistuba, Josif Grabocka
HPO-B: A Large-Scale Reproducible Benchmark for Black-Box HPO based on OpenML
Neural Information Processing Systems, Datasets and Benchmarks Track (NeurIPS 2021) -
Arlind Kadra, Marius Lindauer, Frank Hutter, Josif Grabocka
Well-tuned Simple Nets Excel on Tabular Datasets
Neural Information Processing Systems (NeurIPS 2021) -
Michael Ruchte, Josif Grabocka
Scalable Pareto Front Approximation for Deep Multi-Objective Learning
IEEE International Conference on Data Mining (ICDM 2021) -
Ahmed Rashed, Lars Schmidt-Thieme, Josif Grabocka
A Guided Learning Approach for Item Recommendation via Surrogate Loss Learning
ACM SIGIR Conference on Research and Development in Information Retrieval (SIGIR 2021) -
Martin Wistuba, Josif Grabocka
Few-Shot Bayesian Optimization with Deep Kernel Surrogates
International Conference on Learning Representations (ICLR 2021) -
Hadi S. Jomaa, Lars Schmidt-Thieme, Josif Grabocka
Dataset2vec: Learning dataset meta-features
Journal of Data Mining and Knowledge Discovery (DAMI 2020) -
Rafael R. Drumond, Lukas Brinkmeyer, Josif Grabocka, Lars Schmidt-Thieme
HIDRA: Head Initialization across Dynamic targets for Robust Architectures
SIAM International Conference on Data Mining (SDM 2020) -
Ahmed Rashed, Josif Grabocka, Lars Schmidt-Thieme
Attribute-aware non-linear co-embeddings of graph features
ACM Conference on Recommender Systems (RecSys 2019) -
Ahmed Rashed, Josif Grabocka, Lars Schmidt-Thieme
Multi-Relational Classification via Bayesian Ranked Non-Linear Embeddings
ACM SIGKDD Conference on Knowledge Discovery and Data Mining (KDD 2019)
Alle Publikationen
Publikationsliste von Prof. Dr. Josif Grabocka auf Google Scholar
Team
Du hast Fragen?
Dann wende dich an unser Machine Learning Lab.